Can AI resume screening be Biased?
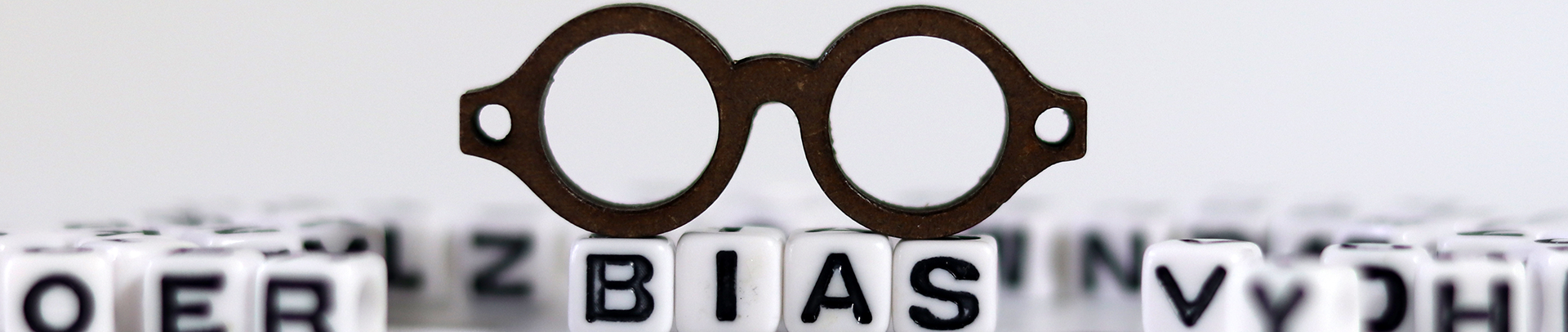
Diversity, equity and inclusion (DE&I) has become an important component of hiring in recent years globally. However, despite the best of intentions, DE&I has had its fair share of criticism for being unable to eliminate recruiting bias. Both conscious and unconscious biases often creep into the recruiting process, resulting in the rejection of deserving candidates. Some common biases include –
Confirmation bias
Recruiters may have preconceived notions or expectations about what an ideal candidate looks like, leading them to selectively focus on information that confirms their beliefs while disregarding contradictory evidence.
Halo/horns effect
The halo effect occurs when a recruiter’s positive impression of a candidate in one area influences their overall evaluation, even if other aspects may not be as strong. Conversely, the horns effect occurs when a negative impression in one area taints the overall evaluation, even if the candidate has strengths in other areas.
Stereotyping bias
Stereotyping bias involves making assumptions about individuals based on characteristics such as gender, race, age, or appearance. Stereotypes can lead to unfair judgments and result in qualified candidates being overlooked or underestimated.
Similarity bias
Recruiters may exhibit a preference for candidates who share similar backgrounds, experiences, or characteristics, leading to a lack of diversity in the hiring process. This bias can perpetuate homogeneity within the organization.
Anchoring bias
Anchoring bias refers to the tendency to rely heavily on initial information when making judgments or decisions. For example, if a candidate’s first impression is negative, recruiters may have difficulty adjusting their perception even if subsequent information suggests the candidate is highly qualified.
Availability bias
Availability bias occurs when recruiters heavily rely on readily available information or personal experiences when evaluating candidates. This can lead to overlooking candidates who may possess relevant skills or qualifications but are not as well-known or easily accessible.
Unconscious bias
Unconscious biases are implicit associations or attitudes that individuals may hold without conscious awareness. These biases can influence decision-making during recruitment without the person realizing it, leading to unfair treatment or discrimination.
Th is probably why companies across the globe have transitioned over to AI-powered recruitment technologies. AI is often credited for mitigating bias in hiring as the technology screens candidates using a large volume of data. AI, through its algorithm, combines various data points and predicts the best-fit candidate for a role or vacancy. The human brain, for all its greatness can’t possibly process information at such a massive scale. While AI objectively assesses the data points and reduces assumptions, mental fatigue and bias that humans often succumb to.
These tools automate almost every step of the recruiting and hiring process, dramatically reducing the burden on HR teams and allowing them the time to focus on the more important aspects of recruitment like interviewing, negotiating etc.
But for all its benefits, are AI-powered recruitment technologies completely unbiased?
The answer is a resounding No.
Back in 2018, Amazon scrapped its AI and machine learning-based recruitment program after it discovered that the algorithm was biased against women. Like most AI-powered recruitment techs, Amazon’s program used to vet candidates by observing the resumes selected by the company in the past 10 years. The majority of which were men, which led the system to deduce male candidates were not only preferred but were also more sought after than female candidates.
Which tell us bias in AI systems during screening can arise from various sources-
Biased training data
AI systems learn from historical data, and if the training data is biased, the AI system can perpetuate those biases. For example, if historical hiring data is biased against certain demographic groups, an AI screening system trained on that data may also discriminate against those groups.
Algorithmic bias
The algorithms used in AI screening can be inherently biased. If the algorithms are not properly designed or tested, they may treat certain groups unfairly. Biases can emerge from the features selected, the weight assigned to those features, or the decision boundaries defined by the algorithm.
Lack of diversity in training data
If the training data used to develop an AI screening system is not diverse and representative of the population, it can lead to biased outcomes. For instance, if the data primarily includes profiles of a particular gender or ethnicity, the AI system may have difficulty accurately assessing candidates from underrepresented groups.
Proxy variables
AI systems sometimes use proxy variables that are correlated with protected attributes (such as race or gender) to make predictions. This can lead to indirect discrimination. For example, if an AI system uses a person’s zip code as a proxy for their socioeconomic status, it may inadvertently discriminate against certain racial or ethnic groups.
Lack of transparency and accountability
The lack of transparency in AI systems can make it difficult to identify and address biases. If the algorithms and decision-making processes are not well-documented or explainable, it can be challenging to understand how bias is introduced or propagated within the system.
Can AI-screening bias be prevented?
Addressing bias in AI screening requires careful attention to data collection and curation, algorithm design and evaluation, and ongoing monitoring and auditing of the system’s performance. It is crucial to have diverse and representative datasets, robust evaluation frameworks, and mechanisms in place to detect and mitigate bias throughout the development and deployment of AI screening systems.
Diverse and representative training data
Ensure that the training data used to develop AI screening systems is diverse, representative, and free from bias. Take measures to collect data from a wide range of sources and demographics, ensuring that it covers various characteristics and backgrounds.
Data preprocessing and cleaning
Thoroughly examine the training data to identify and mitigate any biases present. This may involve removing or reweighting samples that exhibit bias, addressing underrepresented groups, and balancing the dataset to prevent skewed outcomes.
Transparent and explainable algorithms
Use algorithms that are transparent and explainable, allowing users to understand how decisions are made. This promotes accountability and helps in identifying and addressing any potential biases. Avoid using overly complex “black-box” algorithms that are difficult to interpret.
Regular evaluation and auditing
Continuously assess the performance of the AI screening system to detect and correct biases. Conduct regular audits to evaluate the impact of the system on different demographic groups and identify any discrepancies or adverse effects.
Bias testing and fairness metrics
Implement bias testing and fairness metrics to evaluate the system’s outputs across different groups. This involves analyzing how the system’s predictions or decisions vary based on various attributes, such as race, gender, or age. Adjust the algorithms if any disparities or biases are identified.
Ethical guidelines and standards
Establish clear ethical guidelines and standards for the development and deployment of AI screening systems. These guidelines should explicitly address the prevention of bias and discrimination, emphasizing fairness, transparency, and accountability.
Diverse development teams
Foster diverse teams of developers and experts who can bring different perspectives and experiences to the table. This diversity can help identify and challenge biases in the development process and lead to more robust and unbiased AI screening systems.
Ongoing monitoring and user feedback
Continuously monitor the AI screening system’s performance and collect user feedback to identify and rectify any biases or issues that may arise during real-world usage. Actively engage with users and stakeholders to understand their concerns and incorporate their feedback into system improvements.
Completely eliminating bias from AI systems may be challenging, but by implementing these measures, organizations can significantly reduce biases and strive for fair and equitable AI screening processes. Regular updates and improvements based on new research and best practices are also essential to stay up to date with evolving techniques for bias prevention.
Reference
- https://www.forbes.com/sites/forbeshumanresourcescouncil/2021/10/14/understanding-bias-in-ai-enabled-hiring/?sh=45439fcc7b96